The Ultimate Guide to AI Summarization
Artificial intelligence (AI) has revolutionized many industries, and one area where it has made a significant impact is in summarization. Today, we are inundated with information from multiple sources, making it increasingly challenging to sift through the noise and extract the most relevant details. AI summarization offers a solution to this problem by automatically condensing large amounts of text into concise summaries. In this ultimate guide, we will explore the ins and outs of AI summarization, including its definition, science behind it, different types, benefits, challenges and limitations, future trends, and how to choose the right AI summarization tool.
Understanding AI Summarization
Before diving into the depths of AI summarization, let's start with a brief overview of its definition and basics. AI summarization involves using artificial intelligence techniques to generate summaries of textual content. These summaries aim to capture the essence of the original text and provide a concise synopsis for readers.
AI summarization is a fascinating field that combines the power of artificial intelligence with the nuances of natural language processing (NLP). By employing advanced algorithms and models, AI summarization can sift through extensive textual data and distill it into a coherent and concise form, making information more accessible and digestible for users.
Definition and Basics of AI Summarization
AI summarization is a branch of natural language processing (NLP) that leverages various algorithms and models to analyze textual data and extract meaningful information. The goal is to condense a lengthy document into a shorter version while preserving its key points and maintaining coherence.
One of the key challenges in AI summarization is striking a balance between brevity and informativeness. The algorithms must be designed to identify the most crucial information in a text while ensuring that the summary remains coherent and contextually accurate. This delicate dance between compression and retention of information is where the true complexity and ingenuity of AI summarization lie.
The Role of AI in Summarization
AI plays a critical role in the summarization process by automating what was once a time-consuming and labor-intensive task. Through the use of machine learning techniques, AI models can process vast volumes of information, identify important sentences or phrases, and generate concise summaries that capture the essence of the original text.
Furthermore, AI summarization is not just about condensing text; it also involves understanding the underlying meaning and context of the content. By analyzing the relationships between words, phrases, and sentences, AI models can create summaries that go beyond mere extraction of information, providing insights and interpretations that enrich the reader's understanding of the text.
The Science Behind AI Summarization
Now that we have a basic understanding of AI summarization, let's explore the science behind it. Two key areas of study come into play: natural language processing (NLP) and machine learning techniques.
Natural Language Processing and AI Summarization
Natural language processing involves the ability of computers to understand and interpret human language. In the context of AI summarization, NLP algorithms are used to analyze the structure and semantics of text, enabling machines to grasp the salient information and create accurate summaries.
One fascinating aspect of natural language processing is the use of syntactic and semantic analysis to break down sentences into their constituent parts. By understanding the grammar and meaning of each word and phrase, NLP algorithms can identify the most important information in a text. This process involves parsing sentences, tagging parts of speech, and recognizing relationships between words to generate coherent summaries.
Machine Learning Techniques in Summarization
Machine learning algorithms are at the heart of AI summarization. These algorithms are trained on large datasets of text and learn to identify patterns and extract important information. They can differentiate between relevant and irrelevant content, allowing for the creation of accurate and coherent summaries.
One key machine learning technique used in AI summarization is deep learning, specifically through the use of neural networks. These networks are designed to mimic the human brain's ability to learn and adapt. By processing vast amounts of textual data, neural networks can automatically generate summaries by recognizing key phrases, understanding context, and organizing information in a logical manner.
Different Types of AI Summarization
AI summarization can be broadly classified into two main types: extractive summarization and abstractive summarization.
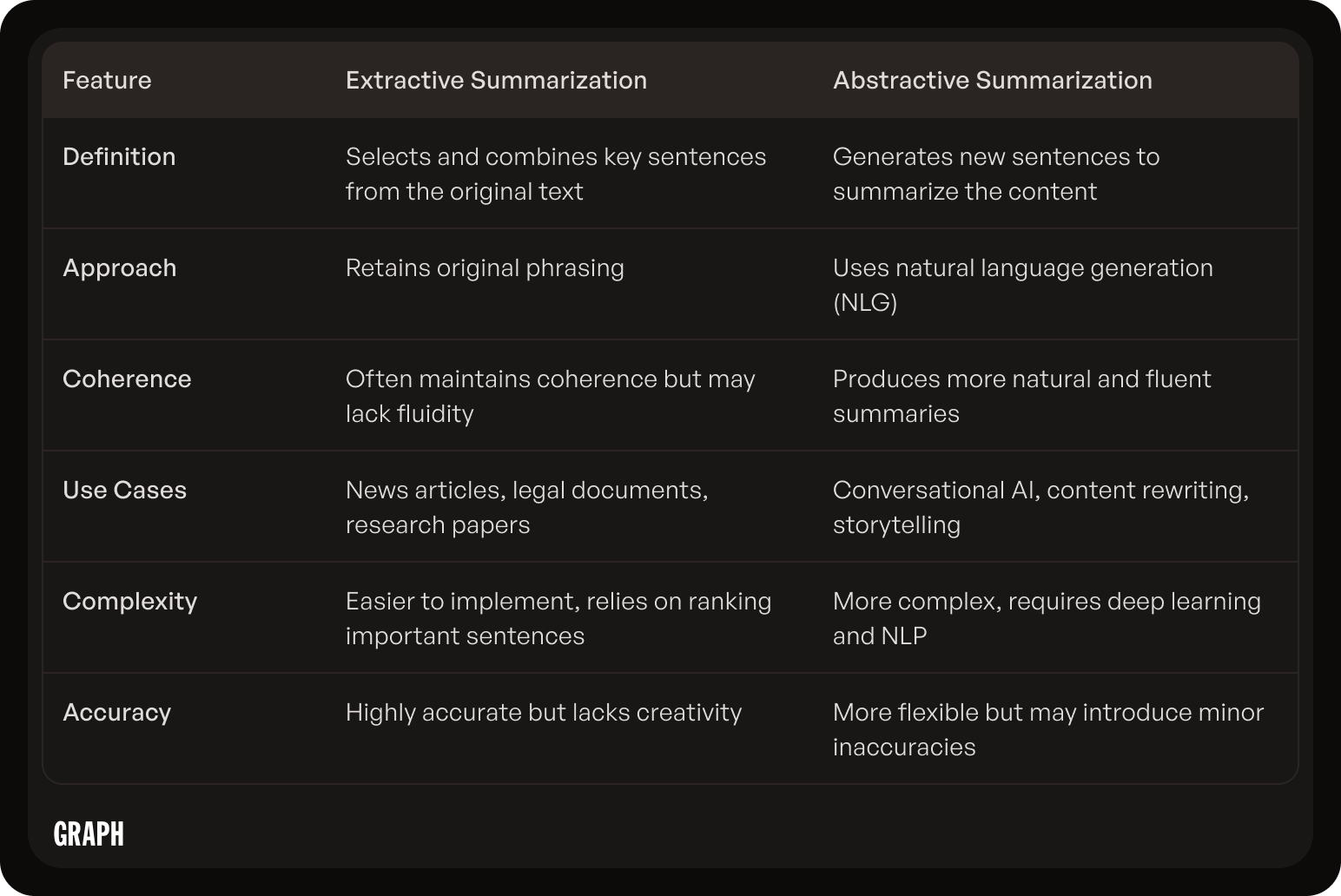
When it comes to AI summarization, there are various techniques and methodologies that can be employed to condense large amounts of text into concise summaries. These methods play a crucial role in information retrieval and text processing tasks, aiding in the extraction of key points and reducing the overall content while preserving its essence.
Extractive Summarization
Extractive summarization involves selecting and combining relevant sentences or phrases from the original text to create a summary. This approach aims to retain the original wording as much as possible and often relies on ranking sentences based on their importance. Extractive summarization is commonly used in news articles and scientific papers.
One of the key challenges in extractive summarization is ensuring that the selected sentences are not only relevant but also flow cohesively to form a coherent summary. This process often involves the use of algorithms that analyze the text's structure and semantics to determine the most salient information to include in the summary.
Abstractive Summarization
Abstractive summarization takes a more creative approach by generating summaries that do not necessarily include excerpts from the original text. Instead, it generates new sentences that capture the essential information. Abstractive summarization leverages advanced language models and techniques like natural language generation (NLG) to produce coherent and concise summaries.
Unlike extractive summarization, abstractive summarization requires a deeper understanding of the text to generate human-like summaries that convey the original meaning in a more condensed form. This approach often involves tasks such as paraphrasing, rephrasing, and restructuring sentences to create a cohesive and informative summary that captures the essence of the source material.
Benefits of AI Summarization
The adoption of AI summarization offers several benefits that can greatly enhance our ability to process and comprehend large amounts of information.
AI summarization technology is revolutionizing the way we handle information overload by condensing lengthy documents into concise summaries that capture the essence of the content. This transformational tool not only saves time but also enhances productivity by allowing users to quickly extract key insights without having to sift through pages of text.
Efficiency and Speed
AI summarization significantly reduces the time and effort required to extract key information from lengthy documents. By automating the summarization process, AI enables us to quickly grasp the main points and focus our attention on areas of interest.
Furthermore, the speed at which AI algorithms can generate summaries is unparalleled, making it an invaluable asset for professionals who need to stay updated on a vast amount of information in a limited amount of time. Whether it's scanning through research papers, news articles, or business reports, AI summarization can provide a quick overview of the most important details.
Accuracy and Consistency
Machine learning algorithms used in AI summarization can analyze and process vast amounts of data with high accuracy. By eliminating human bias and inconsistency, AI-generated summaries provide a more objective and consistent view of the information.
Moreover, the consistency in the quality of summaries produced by AI ensures that users can rely on the information presented to make informed decisions. This level of accuracy not only saves time but also reduces the risk of overlooking critical details that could impact the outcome of a project or decision.
Challenges and Limitations of AI Summarization
While AI summarization offers numerous benefits, it also faces certain challenges and limitations that are important to consider.
One significant challenge in AI summarization is the ability to maintain the tone and style of the original text. AI models may struggle to capture the unique voice of the author, leading to summaries that lack the intended flair or emotion. Researchers are exploring ways to incorporate style transfer techniques into summarization models to address this limitation.
Understanding Context and Nuance
AI models struggle to understand context and nuance in the same way humans do. This can result in summaries that miss important subtleties or fail to capture the overall meaning of the original text. Improving context understanding is a key area of research in AI summarization.
Furthermore, another aspect that adds to the complexity of context understanding is the challenge of dealing with ambiguous language. Words or phrases that have multiple meanings can confuse AI models, leading to inaccurate or misleading summaries. Researchers are exploring the use of contextual embeddings and semantic analysis to enhance the ability of AI systems to disambiguate language.
Handling Complex Information
Complex topics or technical jargon can pose a challenge for AI summarization models. Summarizing such content accurately requires deep domain knowledge and specialized algorithms. Advancements in AI techniques are constantly being made to address this limitation.
In addition to technical challenges, ethical considerations also play a role in the development of AI summarization systems. Ensuring that the summarization process respects privacy, avoids bias, and maintains transparency is crucial for building trust with users. Researchers and developers are actively working on incorporating ethical frameworks and guidelines into AI summarization practices to address these concerns.
Future Trends in AI Summarization
The field of AI summarization is evolving rapidly, with several future trends shaping its development and applications.
As AI technology continues to advance, researchers are exploring the integration of natural language processing with machine learning to enhance the summarization process further. This fusion of technologies holds the promise of creating summarization systems that can not only condense information but also generate human-like summaries with nuanced understanding and interpretation.
Advancements in Technology
Ongoing advancements in AI technology, including the development of more sophisticated algorithms and models, will likely lead to more accurate and contextually-aware summarization systems. These advancements may also enable the creation of multi-modal summaries that incorporate visual and auditory information.
Moreover, the evolution of AI hardware, such as specialized processing units like GPUs and TPUs, is poised to revolutionize the speed and efficiency of summarization tasks. These hardware improvements will empower AI systems to process vast amounts of data in real-time, opening up new possibilities for dynamic and interactive summarization applications.
Potential Applications and Industries
AI summarization has the potential to impact a wide range of industries and applications. From news organizations and content creators to legal and medical professionals, AI-generated summaries can streamline workflows, improve decision-making, and enhance information dissemination.
Furthermore, the integration of AI summarization tools with virtual and augmented reality technologies is on the horizon, offering immersive and personalized summarization experiences. Imagine a future where users can explore complex information landscapes through interactive visual summaries or receive real-time audio briefings tailored to their preferences and context.
Choosing the Right AI Summarization Tool
When selecting an AI summarization tool, there are several factors to consider to ensure you choose the most suitable option for your needs.

One important factor to consider is the requirements of your specific use case. Are you looking for extractive or abstractive summarization? Extractive summarization involves selecting and condensing the most important sentences or phrases from the source text, while abstractive summarization involves generating new sentences that capture the essence of the original text. Understanding the difference between these two approaches and determining which one aligns better with your goals is crucial in making the right choice.
Another factor to consider is the level of accuracy and customization you need. Some AI summarization tools may offer higher accuracy rates, while others may allow for more customization options. Assessing these factors will guide you in selecting the right tool that meets your specific requirements.
Evaluating Performance and Reliability
Before committing to an AI summarization tool, it is important to evaluate its performance and reliability. Look for tools that consistently provide accurate and coherent summaries. One way to assess performance is by considering user reviews. Reading about other users' experiences can give you valuable insights into the tool's strengths and weaknesses.
Additionally, conducting trials can help you gauge the tool's performance firsthand. By testing it with different types of texts and evaluating the quality of the generated summaries, you can make a more informed decision. It is also beneficial to compare multiple options to ensure you choose the tool that best aligns with your needs.
By considering these factors and evaluating the performance and reliability of different AI summarization tools, you can make a well-informed decision that maximizes the benefits of this technology.
In conclusion, AI summarization holds immense potential in helping us navigate the information overload of the digital age. By leveraging the power of artificial intelligence, we can streamline our access to information, improve decision-making, and enhance productivity. Understanding the science behind AI summarization, the different types available, as well as the benefits and limitations, is essential in harnessing its capabilities effectively. As advancements continue to drive the field forward, it is crucial to choose the right AI summarization tool that aligns with your specific requirements. With the assistance of AI summarization, our ability to quickly and accurately distill complex information will be greatly enhanced.